Synthetic data and its future
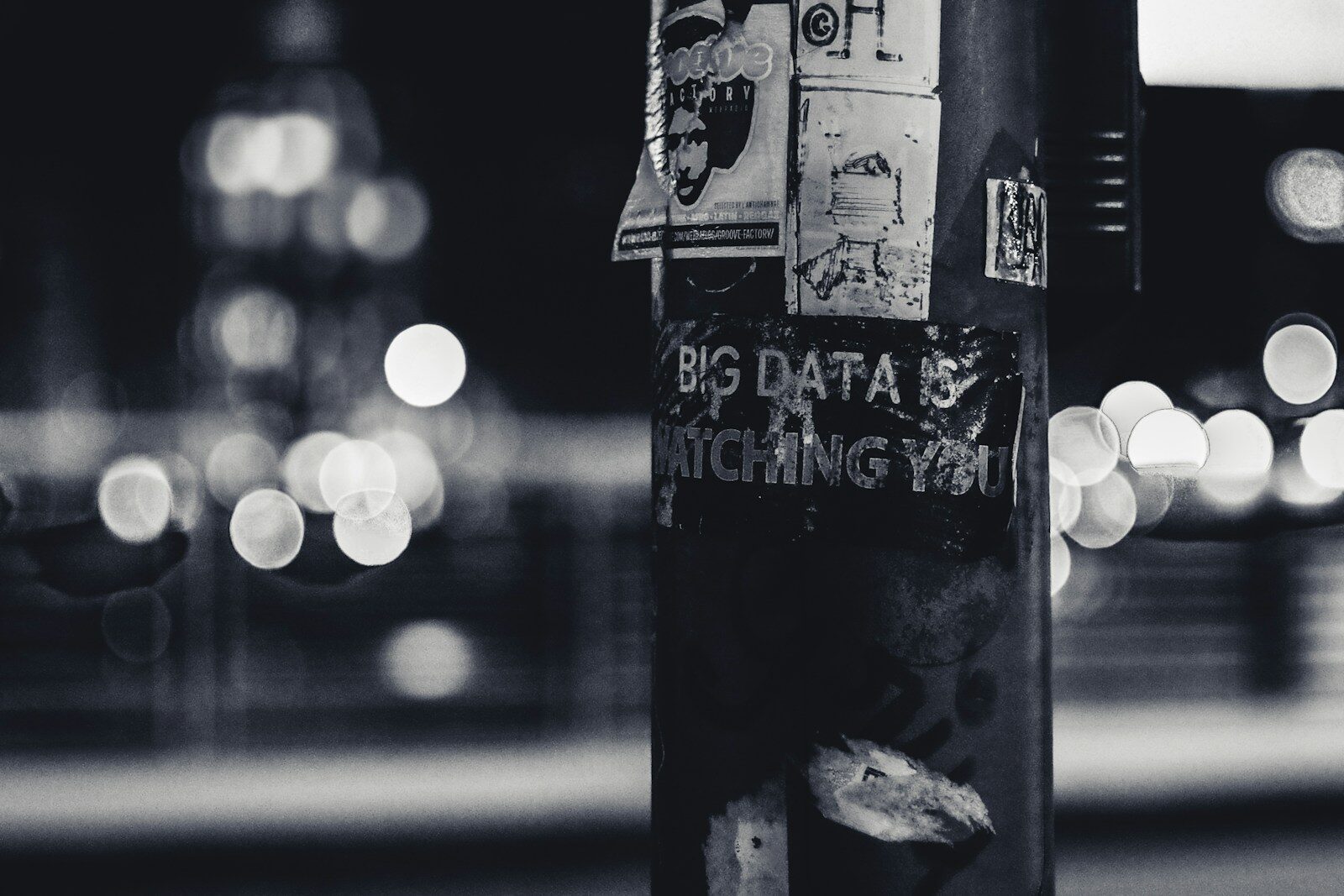
Data is a fundamental, core component of artificial intelligence (AI) and machine learning (ML). However, obtaining large, diverse, and high-quality datasets comes with challenges such as privacy concerns, cost implications, and regulatory constraints. According to Andreas Bartsch, Head of Innovation and Services at PBT Group, this is where synthetic data is stepping in as a game-changer, providing organisations with access to a future where data-driven innovation thrives without compromising privacy or security.
“Synthetic data is algorithmically generated instead of collected from real-world interactions. Unlike traditional test data or anonymised datasets, synthetic data is created to imitate the statistical properties of real data without exposing sensitive information,” says Bartsch.
With AI systems requiring ever-rising volumes of data, Gartner predicts that by 2030, synthetic data will surpass real data in AI model development. This emphasises that synthetic data is a topic that requires attention.
The appeal of synthetic data lies in its ability to overcome the limitations of real-world datasets. One of its key advantages is cost-effective scalability. Generating large datasets is typically costly and laborious, however synthetic data allows companies to create vast, tailored datasets quicker and more affordably.
“Synthetic data also promotes a privacy-first innovation approach that helps businesses to adhere to strict data privacy regulations like GDPR and POPIA. Since synthetic data does not contain personal information, it removes many of the risks associated with handling sensitive real-world data,” he adds.
Another significant advantage is its role in mitigating bias. AI models trained on real-world data often inherit biases present in the original datasets. By carefully engineering synthetic data, companies can create more balanced and representative training sets, improving fairness and reducing unintended biases in AI predictions.
“Furthermore, synthetic data offers customisability. This enables businesses to design datasets that simulate rare or edge-case scenarios that might be underrepresented in real-world data. In doing so, the robustness and adaptability of AI models across various applications are strengthened.”
Real-world applications
Synthetic data is rapidly gaining traction across industries, particularly where data sensitivity and compliance are critical.
For example, in financial services, banks and fintech companies leverage synthetic data to develop fraud detection models and risk assessment algorithms without exposing customer information. The healthcare sector also benefits, as medical research increasingly relies on synthetic patient data to support AI-driven diagnostics while ensuring patient confidentiality.
With autonomous vehicles, self-driving car manufacturers use synthetic data to train AI models on rare but critical driving scenarios that real-world data may not sufficiently capture. Similarly, retail and marketing sectors harness synthetic consumer behaviour data to optimise personalised recommendations without accessing actual user information.
The challenges of synthetic data
“Despite its promise, synthetic data is not a one-size-fits-all solution. One key challenge is ensuring realism. If synthetic data does not accurately reflect real-world complexities, AI models trained on it may struggle with real-world deployment,” adds Bartsch.
Data integrity and trust also remain crucial concerns, as businesses must establish extensive validation methods to ensure that synthetic data maintains the same statistical properties as real data. Additionally, regulatory uncertainty poses a challenge, as evolving data protection laws may still require oversight and transparency in how synthetic data is generated and used.
“Synthetic data represents the next frontier in data science, offering a viable alternative to real-world data with significant advantages. However, like any technological advancement, its success will depend on careful implementation, validation, and a clear understanding of its strengths and limitations,” concludes Bartsch.