Increasing financial opportunities with AI and alternative data
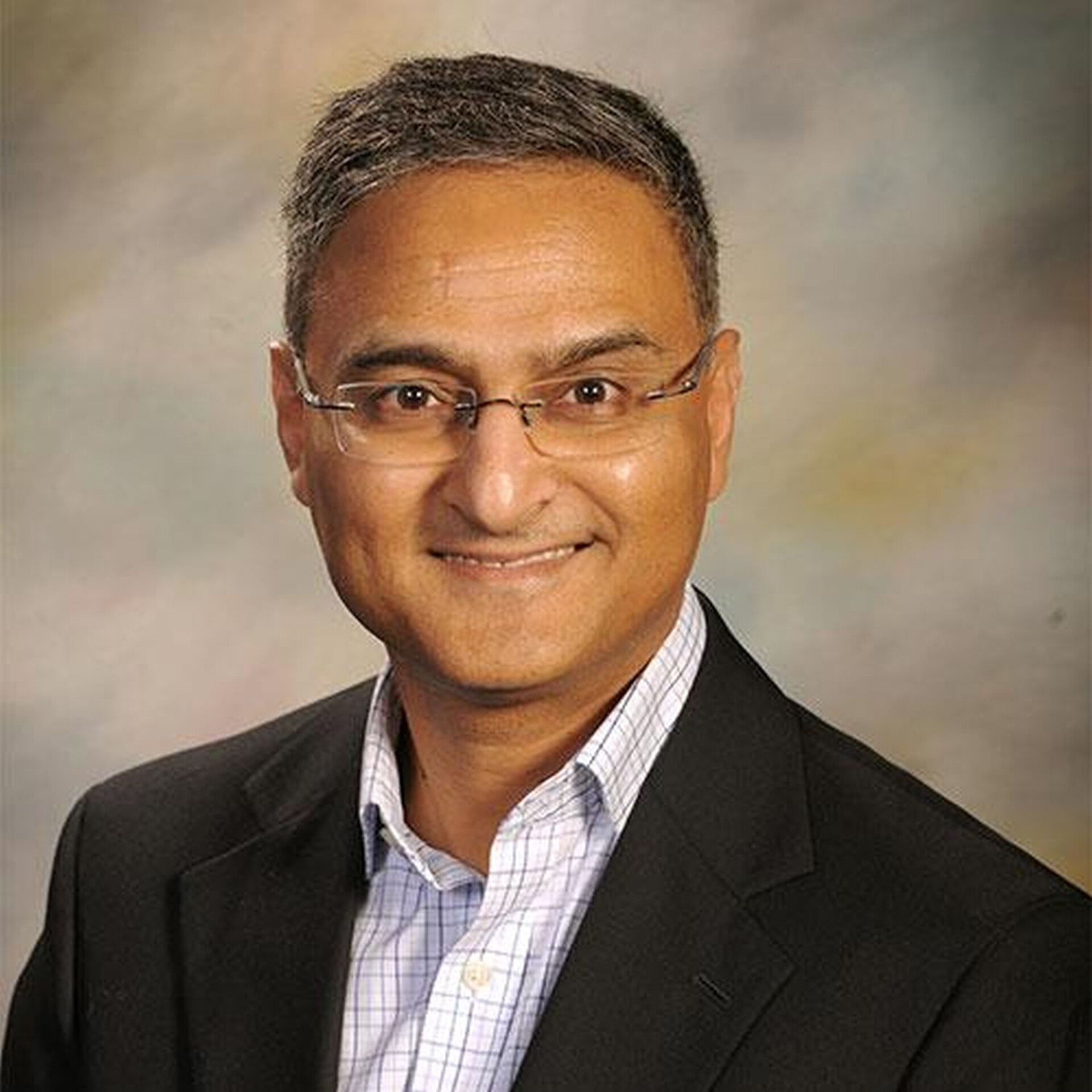
The need for resilience in an evolving financial landscape has never been more important. Economic pressures and digitalisation are reshaping the financial services industry. Technological advancements, such as those resulting from the adoption of artificial intelligence (AI) and machine learning (ML), are providing enterprises with more effective tools to discover insights from ever-expanding and complex data. However, the solutions do not lie solely in technology. Financial services providers must leverage strategic thinking, embrace collaboration, and be committed to responsible innovation.
Consider the issue of financial inclusion. An estimated 1.5 billion people worldwide are still unbanked, leaving them vulnerable to economic shocks and limiting their opportunities for growth. Expanding financial inclusion is not only a moral imperative but also a significant economic opportunity. Inclusive financial systems foster economic growth, reduce inequality, and create more resilient communities.
Using alternative data for financial inclusion
This is where AI and alternative data come into play. Traditional credit risk models rely heavily on established data sources, such as credit bureau scores. While these models have served us well, they exclude individuals and businesses without formal credit histories. Alternative data, such as online behaviour, utility and rent payments, and even mobile phone usage, can provide a measure of creditworthiness for the unbanked and underbanked. By integrating this data with advanced ML techniques, we can build better models and provide an increased level of access to credit for many unable to do so in the past.
AI and ML are better at processing and analysing vast and complex datasets such as digital footprints and transactional history. The more complex ML algorithms can uncover patterns in these datasets that traditional methods might miss. However, not all alternative data is complex. For example, a consumer who regularly pays rent or utility bills on time is creditworthy but is not able to demonstrate this to lenders today.
Reporting such data to the credit bureaus, and incorporating them into risk models and decisions, can expand access to credit for underserved populations while maintaining the underlying integrity of risk assessments.
Of course, data quality and governance remain critical to ensuring that the insights derived are both accurate and fair. In particular, online behaviour data, such as what you like and follow on social media, can be highly correlated to race, religion, nationality, and other prohibited factors. As such, building models on this data requires human oversight as well as clear ethical and legal guidelines to avoid unintended biases that could perpetuate existing inequalities. Ethical AI practices, supported by human oversight, are essential for building trust with consumers and regulators alike.
Addressing housing inequalities
Housing disparities, fuelled by lending inequities, highlight how AI and alternative data can be used to overcome systemic issues. Given the history of South Africa, many communities faced discriminatory practices where access to loans and credit was denied or limited based on racial demographics. This perpetuated economic inequities, limiting home ownership opportunities and generational wealth creation for millions.
By analysing vast datasets using AI, financial institutions can identify patterns of inequity and take corrective action. For instance, a study conducted by the Center for NYC Neighborhoods in partnership with SAS revealed clear disparities in housing outcomes across different neighbourhoods in New York City. Leveraging AI-powered analysis, the study demonstrated how alternative data could pinpoint areas of systemic inequity and inform strategies to address them.
When used responsibly, AI and alternative data can help create a more equitable housing market, fostering long-term economic resilience and inclusivity.
Collaboration and innovation
The future of financial services, in a world of vast complex alternative data and powerful AI algorithms, will be shaped by our ability to harness technology responsibly and collaboratively.
AI and alternative data offer immense potential, but their success depends on collective efforts involving regulators, technology providers, and industry peers. For example, reporting utilities and rent payments to the credit bureaus securely can enable better credit risk assessments within an already trusted framework. Existing regulatory, governance, and ethical practices at lenders must be adapted to new and challenging types of data as well as algorithms that are not as explainable or transparent as previous ones.
Trust is the cornerstone of any financial system. Consumers must trust that lenders are not only sourcing their personal data ethically but also using them in models with a high degree of oversight that results in fair and unbiased decisions.
Fundamentally, the goal is clear. We must collaborate to create a financial ecosystem that is resilient, inclusive, and sustainable. By leveraging the power of AI and alternative data, we can not only address the challenges of today but also build a foundation for a more equitable future.