OpenAI Wants AI to Aid with AI Training for Humans
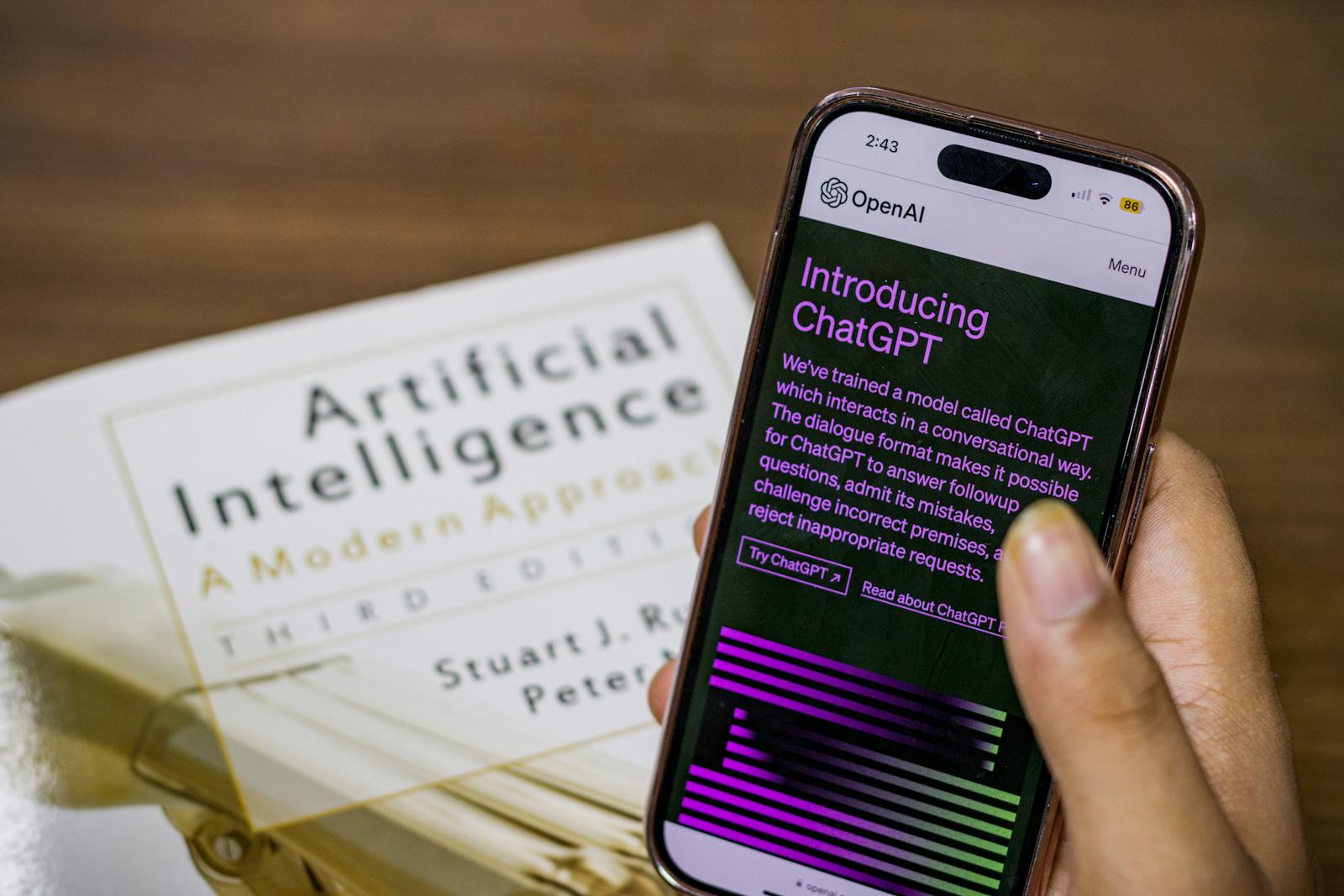
An army of human trainers who provided guidance on good and negative outputs to the artificial intelligence model underlying ChatGPT was one of the main components that made the bot an explosive success. According to OpenAI, incorporating additional AI into the mix to support human trainers may improve the intelligence and dependability of AI assistants.
OpenAI invented the application of reinforcement learning with human feedback, or RLHF, in the development of ChatGPT. This method fine-tunes an AI model based on feedback from human testers to make its output deemed more accurate, coherent, and less offensive. The model’s behavior is determined by an algorithm that is fed by the ratings provided by the trainers. This method has shown to be essential for improving the usefulness and dependability of chatbots as well as keeping them from misbehaving.
“RLHF has some significant limitations, but it does function extremely well,” explains OpenAI researcher Nat McAleese in reference to the latest findings. Human input can be inconsistent, to start. Furthermore, even proficient people may find it challenging to assign a value to exceedingly intricate outputs, such intricate software code. Additionally, a model may be optimized through this approach to yield results that appear persuasive rather than factual.
By optimizing its most potent product, GPT-4, OpenAI created a new model to help human trainers who are in charge of evaluating code. The new model, called CriticGPT, was able to identify faults that human judges missed, according to the business, and its code criticisms were deemed superior by judges 63 percent of the time. In the future, OpenAI plans to investigate expanding the concept outside of the realm of code.
“We’re starting work to integrate this technique into our RLHF chat stack,” McAleese says. He notes that the approach is imperfect, since CriticGPT can also make mistakes by hallucinating, but he adds that the technique could help make OpenAI’s models as well as tools like ChatGPT more accurate by reducing errors in human training. He adds that it might also prove crucial in helping AI models become much smarter, because it may allow humans to help train an AI that exceeds their own abilities. “And as models continue to get better and better, we suspect that people will need more help,” McAleese says.
The new method is just one of several being developed right now to enhance big language models and extract more functionality from them. It’s also a part of the endeavor to guarantee that, even as AI gets more powerful, it acts in ways that are appropriate.
Anthropic, a competitor of OpenAI established by former workers, revealed earlier this month that Claude, its chatbot, has become more proficient due to enhancements made to the model’s training routine and the data it receives. Lately, Anthropic and OpenAI have also bragged about novel approaches to examining AI models to learn how they generate their output and so better prevent undesirable behavior, including lying.
The new method could aid OpenAI in developing increasingly potent AI models while guaranteeing that their output is more reliable and consistent with human values—especially if the business is successful in applying it to domains other than programming.
According to OpenAI, it is currently in the process of training its next big AI model, and it seems that the firm is eager to demonstrate its commitment to assuring proper behavior. This comes after a well-known team devoted to evaluating the long-term hazards associated with artificial intelligence was disbanded. Ilya Sutskever, a former board member and cofounder of the company, co-led the team and momentarily forced CEO Sam Altman out of the company before renouncing and assisting him in regaining control. Since then, a number of those team members have chastised the business for taking unnecessary risks in its haste to create and market potent AI algorithms.
Professor Dylan Hadfield-Menell of MIT, who studies how to align AI, notes that the notion of using AI models to assist in training more potent ones has been around for a while. He claims that “this is a pretty natural development.”
According to Hadfield-Menell, analogous ideas were addressed several years ago by the researchers who invented the methodology employed for RLHF. He thinks its effectiveness and general applicability have still to be determined. “It could result in significant improvements in each person’s abilities and, eventually, serve as a springboard for feedback that is more useful,” he says.