The Sure-Fire to Have Successful AI Projects
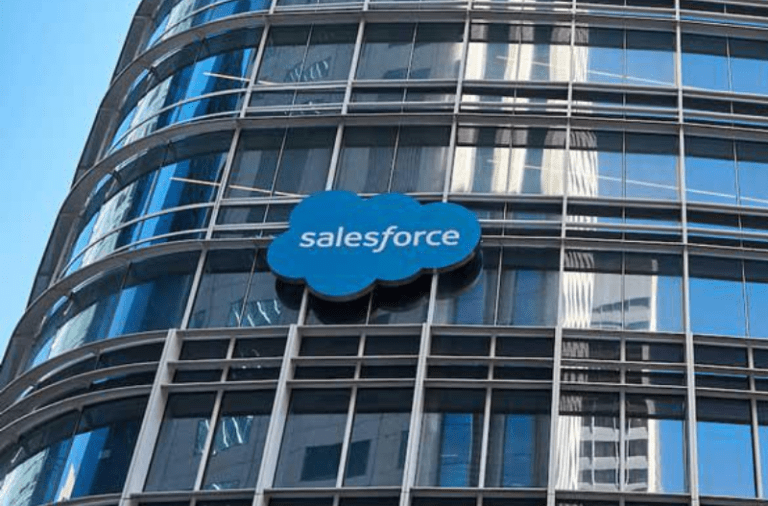
When it comes to selecting the ideal AI project for their firm, business owners frequently rely on having the correct ingredients and knowing how to blend them.
Almost every working AI system today operates according to some set of rules that are encoded in the system and effectively hold everything together.
The ability of their teams to successfully communicate as data scientists, as well as continuously asking oneself a series of questions throughout the development process, is also critical to success.
We can break AI down into ingredients since it can accomplish a wide range of things. When you understand what they are, you can have an understanding of their powers.
The first component is a series of “yes” and “no” questions. For example, if I send you an email, do you intend to open it? These indicate the likelihood that something will occur. This is a question we pose at every level of the AI project.
The second component is numerical forecasting. For example, how long will it take you to pay your bill? Or how long will it take me to repair this person’s refrigerator?
Finally, there are classes. For example, when taking a picture of your team, ask yourself, “Are there people in this picture?” “How many people are there in this photograph?” There are also text categories, which you can see when interacting with a chatbot.
The fourth component is conversions, which involve collecting information and converting it from one format to another. Voice transcription or translation could be used.
Where to Begin
When embarking on an AI project, one of the first things you should ask is, “What data do we have?” And, “What concrete difficulties can it help me solve?”
Consider something that every salesman does as a normal part of their job: categorizing a lead by assigning it a score indicating how probable it is to close. These kind of data sets are a critical source of truth for developing an AI-based project.
People want to do all kinds of things with AI skills, but you can’t if you don’t have the data.
How to Move From a Pilot to a Full-Fledged Rollout
To move the project from the pilot stage to the rollout stage, the first question you must answer is what problem you are attempting to tackle. Is it my intention to prioritize other people’s time? Is it possible that I’m attempting to automate something new? Then you may confirm whether or not you have the data for this project.
The second point to consider is if this is a reasonable goal. Simply said, attempting to automate 100 percent of your customer support enquiries is a recipe for disaster.
However, if 25% of your customer support enquiries are requests to reset a password, and you want to automate it and remove it from your agents’ to-do list, that is a legitimate goal.
Another critical question is, “Can a person accomplish it?” The majority of the time, AI cannot achieve anything that humans cannot.
There are two reasons why AI programs have uncomfortably long pilot phases – and should.
First, you should check to see if it actually works as it should. The context and importance of AI-powered recommendations may vary, from healthcare diagnosis to movie recommendations, but eventually you must offer the reason so your people will believe it.
Second, you must assess the value of the AI solution in comparison to the baseline – human contact. Consider automating customer service inquiries. If your chatbot is unable to answer your clients’ inquiries, you will have disappointed consumers who despise your chatbot and prefer to speak with a human.
Why Do We Need to Stay Rooted?
We know that comprehending and utilizing data sets to inform machine learning algorithms can help to address problems more efficiently. However, intuition plays a crucial role in this process as well.
Consider creating a bespoke prediction for questions such as, “Will my customer pay their bill late or not?”
Data scientists in the AI sector frequently conceive in terms of algorithms or slightly higher level abstractions. What we actually need to do is get into the heads of our clients and convey the answer to the problem in terms that they will understand. This indicates that it’s not simply about providing a recommendation; it’s about advocating the specific component of a project. Furthermore, it is not simply providing a prediction, but directly answering the question, “Are you going to pay your bill or not?”
From here, you must decide what you will do if I make that forecast and give you a probability of the individual paying late.
Finally, teams must remain grounded when deciding what challenges to attempt to address with AI and what resources they have available to assist them. It all boils down to the question of whether or not a person can do it. If they can, AI could be a terrific method to free up a human’s time for more strategic responsibilities.
Article written by By Robin Fisher, Senior Area Vice President, Salesforce Emerging Markets