Graph Neural Networks and Complex Data Representation
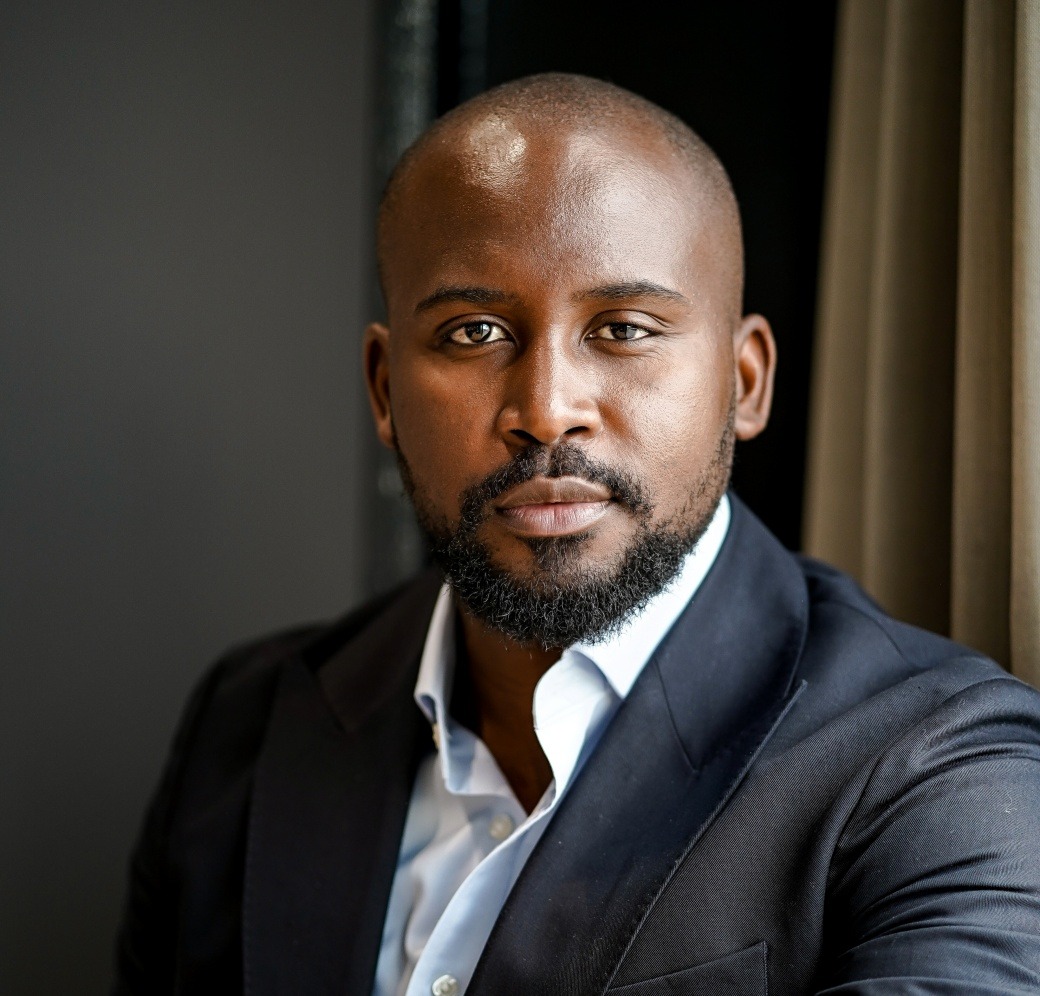
In today’s data-driven world, the capacity to handle and interpret intricate data relationships is more critical than before. Gamaliel Okotie, a senior data scientist with a deep focus on advanced machine learning, has been at the forefront of pioneering methods for complex data representation leveraging Graph Neural Networks. His work in this evolving domain is remoulding industries, from social network analysis to recommendation systems and molecular studies.
Graph Neural Networks offer a sophisticated approach to representing data that traditional methods struggle to handle. Unlike conventional neural networks that operate with fixed-size vectors, Graph Neural Networks succeed in representing data as graphs, collections of nodes and edges that capture relationships and interactions in an explicit form. Gamaliel Okotie has leveraged this robust toolset to explore patterns in different fields.
The power of GNNs lies in their ability to model complex dependencies, such as the links between individuals in a social network or the molecular bonds in chemical compounds. This capability allows Graphical Neural Networks to extend beyond ordinary data processing and venture into reasoning about the structure of the data itself, making them valuable in applications where relationships are key.
One of the most impactful applications of Gamaliel’s work with Graphical Neural Work is in social network analysis. Social networks are inherently robust, filled with multi-dimensional interactions that are often hard to capture with traditional techniques. Graphical Neural Networks, however, gives rooms for the modelling of users as nodes and their relationships including friendships, collaborations, and influences as edges. This provides a deep understanding of network dynamics, identifying influencers, detecting communities, and even predicting future interactions.
By leveraging Graphical Neural Networks, Gamaliel has been able to extract deeper insights into social behaviours, scaling targeted marketing strategies and enhancing user experiences in social platforms. His work showcases how Graphical Neural Networks can scale engagement and recommendation systems, making them highly adaptive to user preferences and behaviours.
Recommendation systems have long been a foundation of online platforms, from e-commerce to streaming services. Custom recommendation models often fall short when dealing with robust user- item relationships. Gamaliel’s integration of Graphical Neural Networks has empowered a more nuanced approach to recommendations, identifying that a user’s preferences are influenced not just by direct interactions but also by indirect relationships within a larger graph.
Through Graphical Neural Networks-based systems, Gamaliel has been able to dramatically scale the accuracy of recommendations by modelling user-item interactions as part of a broader network. This ensures that recommendations are more personalised and invoked, crafting a solid and more intuitive user experience. The utilisation of Graphical Neural Networks in this context has led to innovative breakthroughs in dynamic and context-aware recommendations, making these systems more responsive and effective than ever before.
Beyond social networks and recommendation systems, Gamaliel has expanded the application of Graphical Neural Networks into the field of molecular studies. Molecules, including social networks, are framed as graphs, where atoms are nodes and chemical bonds are edges. Traditional techniques for molecular representation, such as molecular fingerprints, are regularly limited in encapsulating the robustness of molecular interactions. Graphical Neural Network, on the other hand, can represent these interactions more comprehensively, offering new avenues for drug discovery and material science.
Gamaliel’s work in molecular studies has focused on using GNNs to predict the properties of molecules, simulate chemical reactions, and even discover potential new compounds. This has not only increased the speed of research in molecular science but also created a path for more targeted and effective drug design, where predicting molecular behaviour is important.
Gamaliel Okotie’s impact to the field of Graph Neural Networks are advancing the way we understand and use data across various industries His work has proven that Graphical Neural Networks are more than a niche tool, they are a transformative technology that assist us to unravel the opportunity of complex data relationships.
In a society where data complexity continues to transcend, Gamaliel Okotie’s expertise in Graph Neural Networks is pushing the boundaries of what is attainable, ensuring that we remain equipped to navigate and make sense of the diverse relationships that shape our digital and physical environments.