The evolution of data’s ‘AI-dentity’
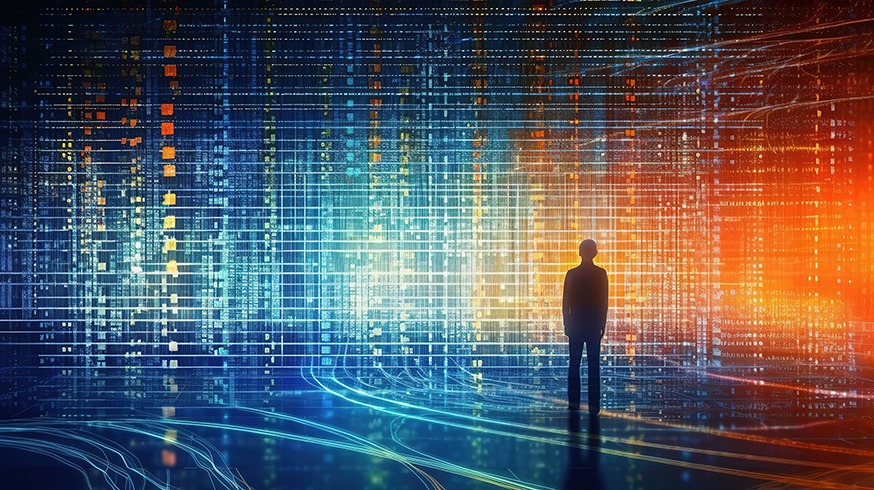
In a time where data drives decision making and innovation, the journey of artificial intelligence (AI) has transformed from a technical concept to a cornerstone of modern business strategies. And today, we’ve reached the point in the evolution of data’s ‘AI-dentity’ that demands careful navigation to ensure that organisations are able to unlock real business value, but also maintain ethical and responsible practices.
Climbing Maslow’s AI hierarchy
Phil Anderson, Sales Manager for Digital Business Solutions at Datacentrix, places significant focus on having the right building blocks in place to manage risk versus deriving business value.
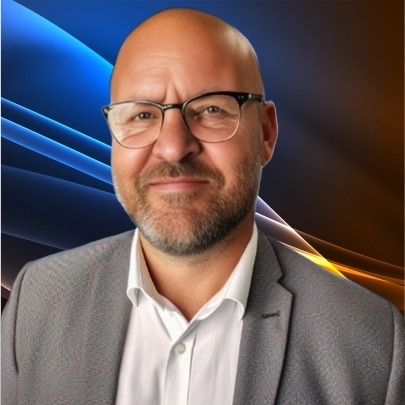
Anderson draws a parallel between Maslow’s hierarchy of needs and a business’s journey with AI, where foundational layers such as responsible and ethical AI policy, robust data management and governance, considered platform choices, and the human factors all need to be considered to form the foundation of sustainable innovation. This is reflected in the current market, where scaling past proof of concept (POC) stage is a challenge for most organisations. Not only is this a problem for business value realisation, but also presents real and tangible risk to reputation and legislative compliance.
“Before deploying advanced AI capabilities, companies must ensure ethical and transparent practices, balancing innovation with the human impact on customers and employees. There is a responsibility on all of us to ensure that AI enhances the human experience, and is deployed to generate outcomes without unfair bias.”
Anderson further highlights the risks of unfettered AI advancements, referencing the rise of publicly available AI tools that operate without sufficient guardrails and protections. “It’s not just the dark web anymore; AI’s risks are surfacing in public platforms every day. This makes it crucial for African organisations to adopt a robust stance on ethical AI now, ensuring their risks are managed.”
The unsung hero – data management
Data management and governance often take a backseat in AI conversations, yet they are critical for success. Arno Hanekom, Digital Strategist for Digital Business Solutions at Datacentrix, underscores this point: “In the past few months alone, as Datacentrix, we’ve engaged in over 80 discussions with clients on AI, and rolled out a number of proof-of-concept projects. Everyone wants AI, but few are prepared to address the personal and invasive nature of data management.”
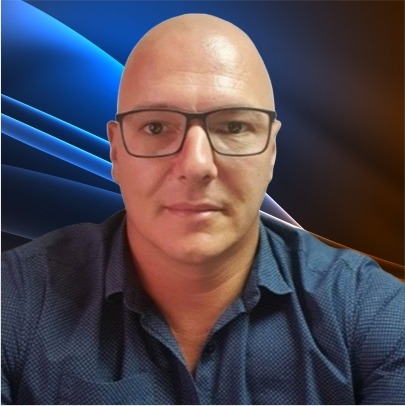
However, this process can be simplified by taking a stepwise approach, managing metadata and creating scalable models. “The goal here is to make data governance less daunting while building collaborative models with our clients,” says Hanekom.
Choosing the right platform
Companies are facing a choice between product-specific AI extensions and deploying enterprise-wide AI platforms to cover wider scope challenges. While the former is quick to implement and has pre-defined use cases, it lacks the scalability of the latter.
“Enterprise-wide platforms provide the flexibility to govern, manage and exploit data across the organisation, ensuring many different use cases can be managed to the same standards,” explains Anderson. “The key is to carefully evaluate use cases and make smart choices on the chosen platform on which to deploy. In today’s market, OEM licencing models are moving and changing every day so it is important to have a partner that can help you dissect this landscape and cut through the complexity.”
The human element in AI
Despite its technical underpinnings, AI remains a profoundly human endeavour. “Our clients value trust and credibility,” Hanekom notes. “Technology is effectively just a byproduct of effective communication and collaboration. Thus, companies should foster a culture of critical thinking, problem-solving and cross-functional collaboration to ensure that their AI implementations resonate with human values and organisational goals.”
Scaling MVPs to business value
One of the persistent challenges in AI adoption is scaling minimum viable products (MVPs) and POCs into enterprise-wide solutions. “Why do so many AI projects falter at the scaling stage?” Anderson asks. “To get to scale, companies must not be too easily sold on the shiny outcomes being presented in the demonstrations and POCs they are being bombarded with today. The key is to understand and address early the impact on organisational policy, process and the people in the function being affected, as these are the key factors that prevent scaling and full value realisation.
“By addressing these organisational barriers, for example outdated policies and inconsistent governance practices, right at the outset, then the path to scale becomes significantly smoother.”
A future powered by AI-dentity
“The evolution of data’s AI-dentity is as much about technological prowess as it is about ethical stewardship and strategic foresight. At Datacentrix, we are committed to guiding our clients on this journey, ensuring that their AI solutions are as responsible as they are transformative,” Hanekom concludes.
For more information on Datacentrix’s approach to AI, please visit https://www.datacentrix.co.za/artificial-intelligence–analytics.html