Meta Unveils the World’s Fastest Supercomputer, AI Research SuperCluster
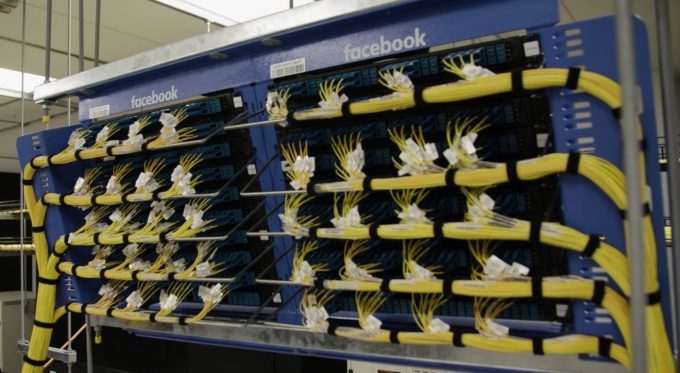
There’s a global race to develop the world’s largest, most powerful computers, and Meta (AKA Facebook) is ready to join the fray with the “AI Research SuperCluster,” or RSC.
When fully operational, it might be one of the top ten fastest supercomputers in the world, used for the huge number-crunching required for language and computer vision modeling.
Large AI models, the most well-known of which is OpenAI’s GPT-3, aren’t built on laptops and PCs; they’re the result of weeks and months of persistent calculations by high-performance computing systems that exceed even the most cutting-edge gaming setup.
And the faster you can complete a model’s training process, the faster you can test it and create a new and improved one. When training times are measured in months, this is quite important.
RSC is now operational, and the company’s researchers are putting it to use… It must be emphasized that the data is user-generated, however Meta was cautious to state that it is encrypted until training time and that the entire facility is separated from the wider internet.
The RSC team should be pleased of themselves for pulling this out nearly entirely remotely – supercomputers are surprisingly physical structures, with basic factors like heat, cabling, and connection affecting performance and design.
Exabytes of storage appear to be large enough digitally, but they must also exist somewhere, on site and accessible in a microsecond’s notice. (Pure Storage is also pleased with the system they devised for this.)
RSC presently has 760 Nvidia DGX A100 workstations with a total of 6,080 GPUs, putting it roughly in competition with Perlmutter at Lawrence Berkeley National Lab, according to Meta. According to the long-running rating site Top 500, this is the fifth most powerful supercomputer in operation right now. (By far the most popular in Japan is Fugaku, in case you were wondering.)
That may alter as the corporation continues to develop the technology. They intend to make it around three times more powerful in the end, which would put it in contention for third place.
There’s a possible caveat there. Systems such as the second-place Summit at Lawrence Livermore National Lab are used for research applications when precision is critical. When modelling molecules in a region of the Earth’s atmosphere to unprecedented detail levels, every calculation must be performed to a large number of decimal points. As a result, the calculations are more computationally costly.
Meta explained that AI applications don’t require the same level of precision because the results don’t depend on that thousandth of a percent — inference operations produce things like “90 percent certainty this is a cat,” and whether that number was 89 percent or 91 percent wouldn’t make a big difference. The challenge is reaching 90% certainty for a million objects or phrases rather than a hundred.
It’s an oversimplification, but the effect is that RSC can get more FLOP/s (floating point operations per second) per core than other, more precision-oriented systems while running TensorFloat-32 math mode. In this scenario, it’s up to 1,895,000 teraFLOP/s, or 1.9 exaFLOP/s, which is four times faster than Fugaku’s.
Does it make a difference? And, if so, who? If it matters to anyone, it might matter to the Top 500, therefore I’ve inquired if they have any thoughts on it. But that doesn’t change the fact that RSC will be among the world’s fastest computers, possibly the fastest run by a private enterprise for its own reasons.