Generative AI may be creating more work than it saves
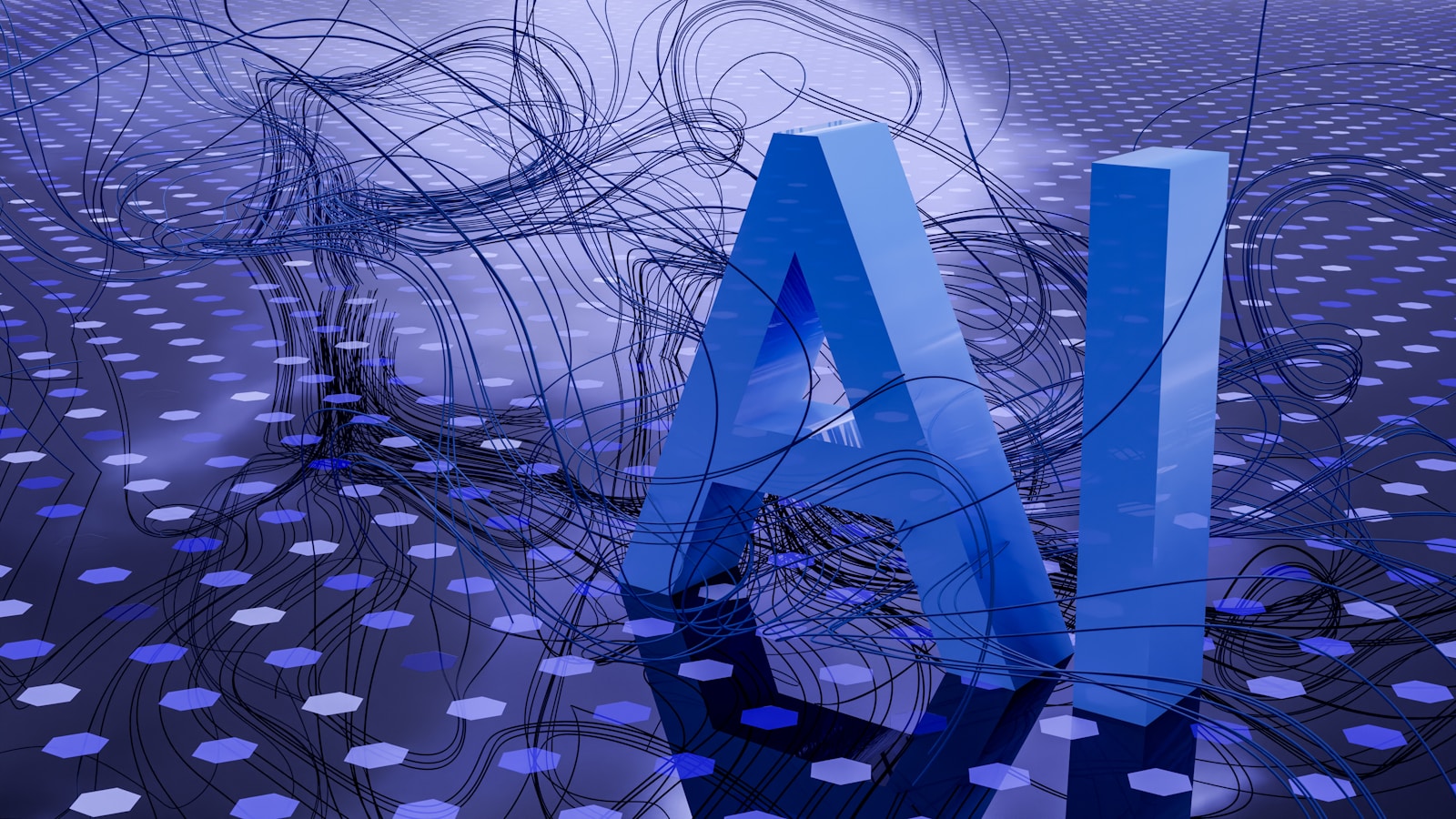
Everyone agrees that using generative artificial intelligence (AI) techniques may increase productivity and save workers time. However, even if these technologies make it simple to run code or generate reports rapidly, the backend labor required to create and maintain large language models (LLMs) may outweigh the labor savings up front. Furthermore, a lot of activities do not absolutely need AI’s power when conventional automation will suffice.
That’s what University of Pennsylvania Wharton School business professor Peter Cappelli said at a recent MIT event. When combined, generative AI and LLMs might make people’s jobs harder rather than easier. Implementing LLMs is challenging, and according to Cappelli, “it turns out there are many things generative AI could do that we don’t really need to do.”
Despite the hoopla around AI as a game-changing technology, he noted that “projections from the tech side are often spectacularly wrong.” “In fact, most of the technology forecasts about work have been wrong over time.” He claimed that the impending wave of autonomous vehicles, which was foreseen in 2018, is an illustration of optimistic predictions that haven’t materialized.
The finer points of technological development can sometimes trip up grandiose plans. Autonomous vehicle proponents focused on what “driverless trucks could do, rather than what needs to be done, and what is required for clearing regulations — the insurance issues, the software issues, and all those issues.” In addition, Cappelli stated: “If you look at their actual work, truck drivers do lots of things other than just driving trucks, even on long-haul trucking.”
The use of generative AI to business and software development is comparable. Developers “spend a majority of their time doing things that don’t have anything to do with computer programming,” he stated. They are engaging in conversations, settling financial matters, and other such activities. Not everything that is done on the programming side is truly programming.”
While innovation has exciting technological potential, practicalities often impede its widespread adoption. Any labor-saving and productivity gains from generative AI may be offset by the backend effort required to create and maintain LLMs and algorithms.
According to Cappelli, “generative and operational AI both generate new work.” “People have to arrange resources, maintain databases, and deal with issues like veracity and conflicting reports, among other things. Many new duties will result from it, and someone will need to complete them.”
He claimed that even though operational AI has been around for a while, it is still being refined. The application of numerical machine learning has been notably lacking. Questions about database administration have played a factor in this. The mere act of compiling the data for analysis requires a significant amount of work. Data is frequently stored in silos across several organizations, which makes it challenging to combine politically as well as technically.”
Cappelli cites several issues in the move toward generative AI and LLMs that must be overcome:
- Addressing a problem/opportunity with generative AI/LLMs may be overkill – “There are lots of things that large language models can do that probably don’t need doing,” he stated. For example, business correspondence is seen as a use case, but most work is done through form letters and rote automation already. Add the fact that “a form letter has already been cleared by lawyers, and anything written by large language models has probably got to be seen by a lawyer. And that is not going to be any kind of a time saver.”
- It will get more costly to replace rote automation with AI – “It’s not so clear that large language models are going to be as cheap as they are now,” Cappelli warned. “As more people use them, computer space has to go up, electricity demands alone are big. Somebody’s got to pay for it.”
- People are needed to validate generative AI output – Generative AI reports or outputs may be fine for relatively simple things such as emails, but for more complex reporting or undertakings, there needs to be validation that everything is accurate. “If you’re going to use it for something important, you better be sure that it’s right. And how are you going to know if it’s right? Well, it helps to have an expert; somebody who can independently validate and knows something about the topic. To look for hallucinations or quirky outcomes, and that it is up-to-date. Some people say you could use other large language models to assess that, but it’s more a reliability issue than a validity issue. We have to check it somehow, and this is not necessarily easy or cheap to do.”
- Generative AI will drown us in too much and sometimes contradictory information – “Because it’s pretty easy to generate reports and output, you’re going to get more responses,” Cappelli said. Also, an LLM may even deliver different responses for the same prompt. “This is a reliability issue — what would you do with your report? You generate one that makes your division look better, and you give that to the boss.” Plus, he cautioned: “Even the people who build these models can’t tell you those answers in any clear-cut way. Are we going to drown people with adjudicating the differences in these outputs?”
- People still prefer to make decisions based on gut feelings or personal preferences – This issue will be tough for machines to overcome. Organizations may invest large sums of money in building and managing LLMs for roles, such as picking job candidates, but study after study shows people tend to hire people they like, versus what the analytics conclude, said Cappelli. “Machine learning could already do that for us. If you built the model, you would find that your line managers who are already making the decisions don’t want to use it. Another example of ‘if you build it, they won’t necessarily come.'”
Cappelli proposed that sorting through data storage and providing analysis to help decision-making processes is the most practical use of generative AI in the near future. “We are washing data right now that we haven’t been able to analyze ourselves,” he stated. “It’s going to be way better at doing that than we are,” he stated. “Someone’s got to worry about guardrails and data pollution issues” in addition to database management.