Harnessing Data Science and Machine Learning for Hyper-Personalized Customer Journeys
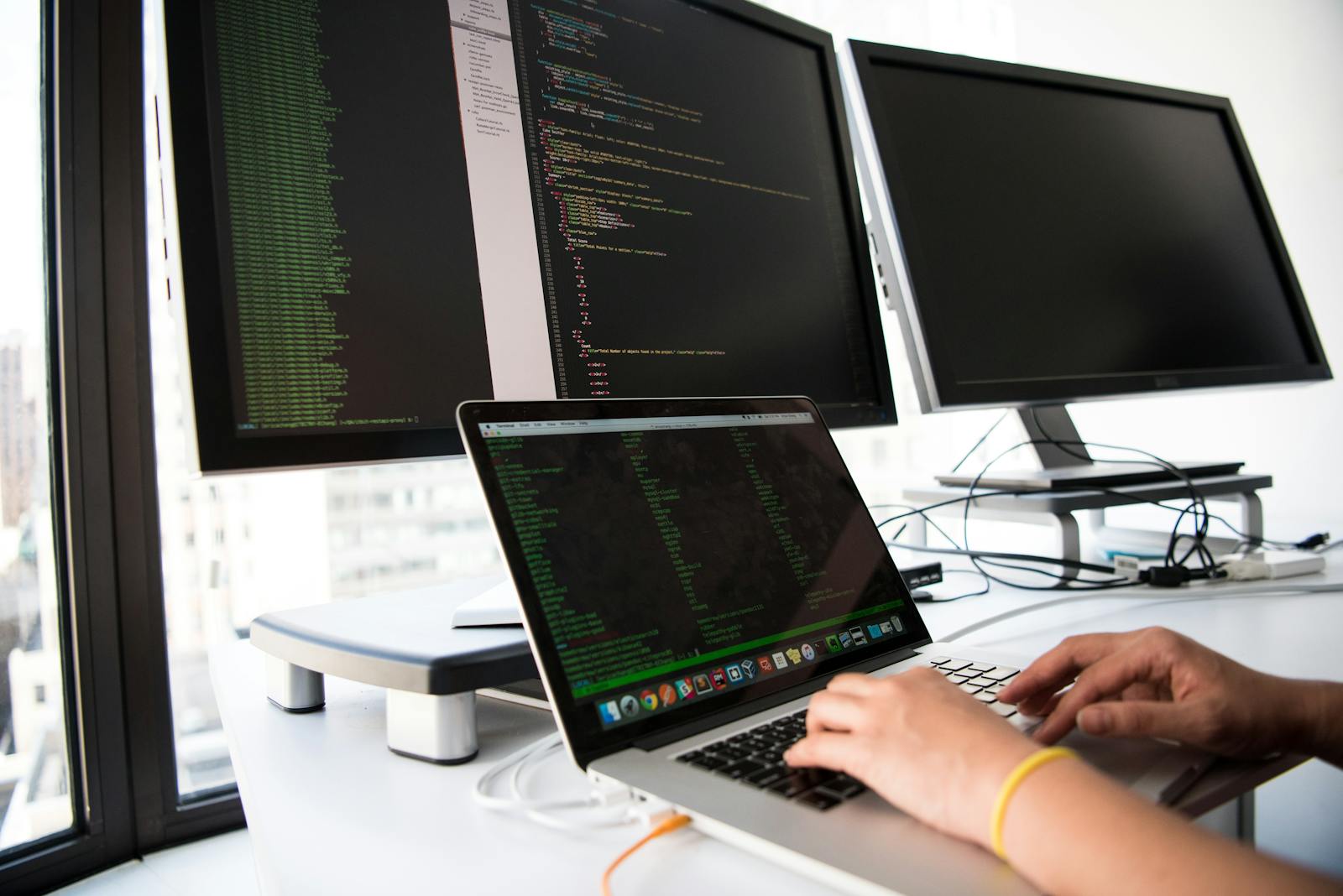
In recent times, when consumers are inundated with options and information, creating a personalized customer experience is no longer just a nice-to-have; it’s a competitive necessity. Businesses, particularly in 1ntech, tech product sales, and OEMs, must go beyond the traditional approaches of mass marketing and one-size-1ts-all services.
The answer lies in harnessing the power of data science and machine learning to deliver hyper-personalized customer journeys—experiences so 1nely tuned and predictive that they not only meet customers’ current needs but anticipate their future desires.
This isn’t about just pushing targeted ads or recommending products that are “similar” to previous purchases. It’s about developing a deep, algorithm-driven understanding of each customer’s behavior, preferences, and lifecycle to oIer solutions, services, and products that feel tailor-made. It’s about seeing the bigger picture and being proactive rather than reactive.
Let’s dive into how data science and machine learning (ML) create these hyper-personalized customer journeys, and why it’s the ultimate game-changer for tech enthusiasts and business strategists alike.
The Evolution from Mass Marketing to Micro-Moments
In the early days of the digital revolution, marketing was largely about broadcasting a message to as many people as possible. Think of it as throwing a wide net and hoping to catch a few 1sh. This approach worked for a while, but as digital ecosystems became more sophisticated, consumers began to demand more. The modern consumer doesn’t want to be bombarded with generic oIers; they want businesses to understand their needs and preferences on a granular level.
Enter data science and machine learning—two forces that transformed the traditional sales funnel into a dynamic, responsive model. With these technologies, businesses can collect, analyze, and interpret vast amounts of data, revealing individual customer preferences and behaviors. This is where hyper-personalization starts: understanding that each customer is unique, with distinct needs, values, and triggers.
Data scientists and engineers work to unlock the power of this data, using ML algorithms to map out customer journeys with micro-moments in mind—those critical points when a consumer is most receptive to engagement.
The Data-Driven Anatomy of a Hyper-Personalized Customer Journey
A successful hyper-personalized journey isn’t built overnight. It requires a robust data architecture, the right algorithms, and constant iterations based on real-time data. Let’s break down the key components:
a. Data Collection & Aggregation
To understand customers deeply, you need data—lots of it. Today’s businesses have access to a treasure trove of data points: purchase history, browsing behavior, mobile interactions, social media activity, geolocation, and more. Data engineers play a critical role here, ensuring that data is collected accurately and stored in a structured manner within data lakes or warehouses. APIs, sensors, and CRM systems are integrated to provide a 360-degree view of each customer.
b. Data Modeling & Machine Learning Algorithms
Once the data is aggregated, it’s time for data scientists to step in. They build predictive models using advanced ML algorithms that analyze past customer behaviors and predict future actions. For example, a 1ntech company can use these models to identify when a customer might need a loan based on changes in their transaction patterns. In the world of OEM sales, machine learning can anticipate when a customer’s tech device might need an upgrade or suggest the best accessories based on usage patterns.
c. Real-Time Personalization
Imagine a customer browsing through a tech store’s online platform. With the help of machine learning, the platform doesn’t just show them generic tech gadgets; it displays products based on their previous browsing history, purchase patterns, and even current online activity in real time. If the user often searches for gaming laptops, the algorithm doesn’t just suggest any gaming laptop; it recommends the model most suited to their preference—perhaps one that aligns with their past purchases or reviews they’ve read. This is the power of real-time personalization enabled by ML.
Fintech and the Hyper-Personalized Experience
Let’s take a real-world scenario to illustrate how data science and machine learning craft these hyper-personalized experiences in 1ntech.
The Scenario: Meet Sarah, a 34-year-old freelance digital marketer living in Nairobi. She has been using a ?ntech app for the past two years to manage her ?nances—everything from budgeting to investment tracking. Recently, Sarah’s spending patterns show an increase in her online purchases related to travel, indicating that she’s planning a vacation. Her account activity also shows she’s transferring funds to her savings account more frequently, signaling that she might be saving for something signi?cant.
The ?ntech app’s data science engine detects these trends and, powered by machine learning models, predicts that Sarah may bene?t from travel insurance or a specialized savings plan tailored to vacations. Instead of waiting for Sarah to search for these options herself, the app sends her a push noti?cation with a personalized recommendation:
“Hi Sarah, planning a trip soon? We’ve curated a special travel savings plan just for you with a 5% bonus for every milestone you reach. Click to learn more.”
This is hyper-personalization in action. The app not only identi?ed Sarah’s need before she expressed it but also made an o?er relevant to her lifestyle and goals. This seamless integration of predictive insights into the customer’s journey builds loyalty, drives engagement, and, ultimately, enhances retention.
Beyond Recommendations: Anticipating Needs
The beauty of data science and machine learning lies not just in responding to customer actions but anticipating needs before they arise. Here’s how it goes beyond recommendations:
a. Churn Prediction Models
For businesses, retaining customers is as important as acquiring them. With churn prediction models, companies can identify users who might be on the brink of leaving based on speci1c patterns (e.g., reduced activity, negative feedback, or app uninstalls). By recognizing these signs early, the system can trigger targeted oIers or personalized messages to re-engage the customer.
b. Dynamic Pricing and O?ers
Machine learning algorithms also make dynamic pricing a reality. For example, in tech product sales, an algorithm can adjust prices based on a customer’s purchase history, preferred price range, or even the time they are most likely to shop. It can oIer discounts on products that align with their browsing patterns or suggest bundles based on their preferences.
c. Hyper-Targeted Content Delivery
Content is king, but context is queen. For OEMs and tech distribution channels, content delivery systems powered by machine learning can analyze user interactions and deliver hyper-relevant content. If a customer has been researching mobile tech, the system might send them articles comparing the latest smartphone models or a personalized video demo tailored to their preferences.
The Future: Integrating AI, IoT, and Blockchain for Hyper-Personalization
Hyper-personalization is just the beginning. As technologies like arti1cial intelligence (AI), the Internet of Things (IoT), and blockchain mature, they’ll bring even more transformative possibilities:
- AI-Powered Customer Support: Imagine AI bots that not only respond to customer queries but also understand the emotional tone, providing responses that match the customer’s mood and preferences.
- IoT for Contextual Personalization: IoT devices can track how users interact with physical products, sending valuable data back to businesses. This means product recommendations could be based not only on online behavior but also on real-world usage.
- Blockchain for Secure and Transparent Personalization: Blockchain ensures that while data is used for hyper-personalization, it remains secure, giving customers control over what they choose to share.
The Bigger Picture
The world of hyper-personalization is about understanding that customers are more than data points—they are individuals with evolving needs and preferences. As a tech enthusiast or professional, embracing this holistic view and leveraging the power of data science and machine learning can transform your business and set you apart in a crowded marketplace.
The future belongs to those who can build customer journeys that not only respond but anticipate, adapt, and engage in a way that feels personal and intentional. Are you ready to utilize the power of hyper-personalization and lead the charge? The data is in your hands—now it’s time to act.
About the Author
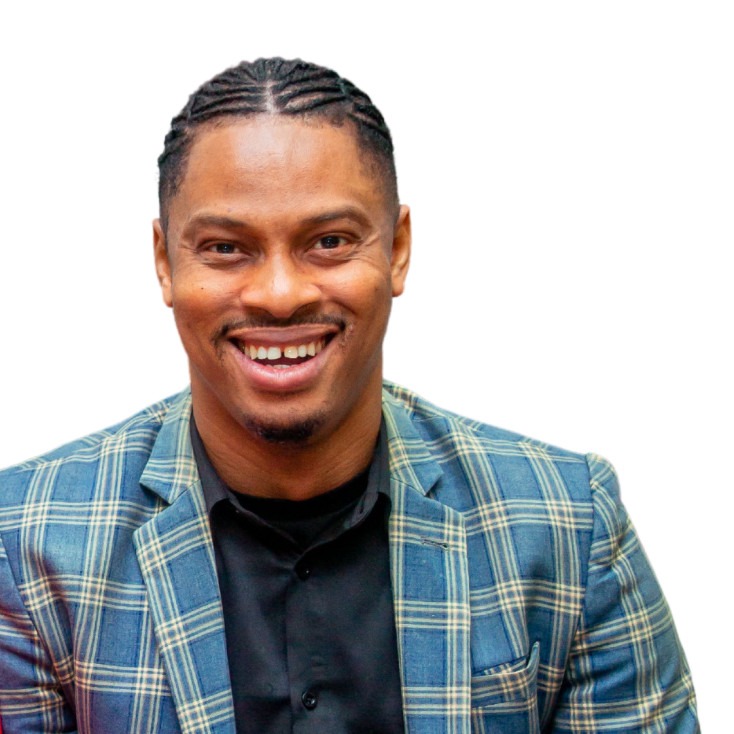
Peter Kwakpovwe is a distinguished Data Scientist and business leader based in the UK. As a certified Scrum Product Owner (CSPO) and a champion of data transformation, he has a proven track record of leading successful business transformations through the strategic application of data, finance, and technology.
With over 12 years of experience in various managerial roles, Peter has been instrumental in building digital products and deriving actionable data insights within the Fintech sector and other digital enterprises. His notable achievements span revenue growth, operational efficiency, business development, and product management, earning him numerous awards and recognition in digital media.
Peter’s expertise encompasses product requirement elicitation, business process re-engineering, data analysis, change management, and the development of digital adoption roadmaps.
He is particularly passionate about creating machine learning models that optimize operations, developing impactful digital products that enhance customer engagement, and extracting meaningful insights from data to drive strategic planning and development.