Building a data governance framework for AI integration
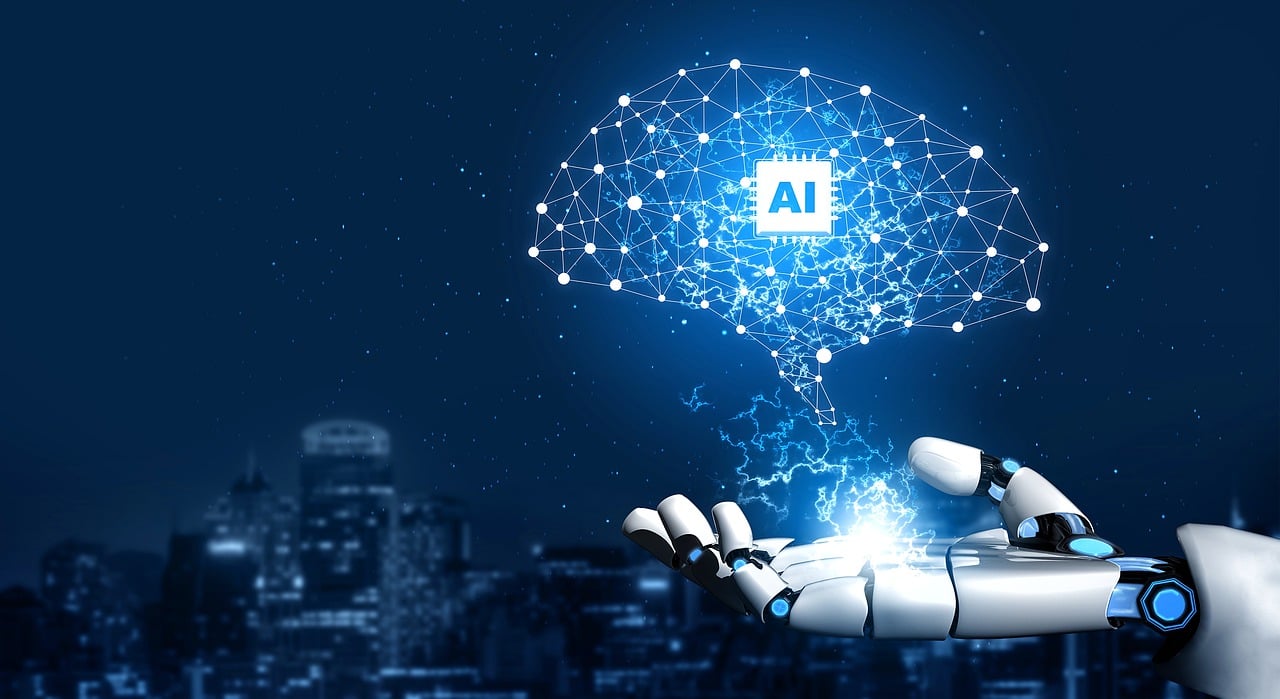
Artificial intelligence (AI) is transforming how companies across industry sectors and geographies govern data. This means that updating data governance frameworks to reflect AI integration is critical for business success and adherence to regulatory requirements. With AI becoming intertwined with data-driven processes, ensuring rigorous standards when it comes to data quality, ethical usage, and privacy protection are non-negotiable. In this piece, PBT Group discusses the key elements of a data governance framework that can effectively support AI integration.
Given how AI is reliant on large datasets, putting in place comprehensive data governance practices should be an essential starting point.
“AI models need high-quality, well-labelled data to deliver accurate outcomes,” says Petrus Keyter, Data Governance Consultant at PBT Group. “Ensuring data accuracy, completeness, and consistency is vital, as any degradation in quality can undermine AI performance.”
To address these needs, data governance frameworks must evolve to include processes for ongoing data validation, quality checks, and error correction. Furthermore, the ethical challenges of AI integration also play a significant role in shaping data governance. AI’s capacity for complex decision-making raises concerns when it comes to bias and fairness.
“Data governance must include ethical guidelines to prevent unintended biases. Compliance with regulations like POPIA and GDPR is also critical to ensure transparency and accountability in AI’s decision-making processes. Regular audits and stringent data usage protocols can help companies align their AI practices both with legal standards as well as customers’ expectations,” adds Keyter.
Additionally, data security and privacy are also vital considerations. AI models often handle sensitive data, increasing the risk of data breaches, unauthorised access, and misuse.
“Implementing a detailed security framework in this regard is essential,” Keyter emphasises. “Data encryption, access control, and anonymisation are necessary to protect sensitive information and maintain trust.”
The unique requirements of AI
Creating an AI-compatible data governance framework means going beyond traditional data management practices. Data quality standards must be even higher, as AI applications require clean, consistent data for optimal performance.
“AI systems benefit from real-time data quality monitoring. Data drift detection tools help maintain these standards over time. Regular assessments ensure that AI systems rely on accurate and relevant data, a foundational element of successful AI integration,” says Keyter.
Another important consideration is understanding the full journey of data, including its origination and lineage. For AI to function transparently and accountably, companies must track the data flow from its source to its transformation and eventual use in AI applications.
“This transparency is crucial for regulatory compliance and troubleshooting. Data lineage tools allow us to trace issues back to their source, making adjustments easier and enhancing the reliability of AI-driven outcomes,” says Keyter.
AI also introduces the need to actively address biases in data. Without careful oversight, AI models can perpetuate existing biases in their training data, leading to unfair results.
“Data governance frameworks must incorporate checks for bias, ensuring fair and ethical AI use. Through regular data audits and diverse data sampling, businesses can reduce biases and create more balanced AI models.”
The sensitive nature of data often used in AI means that privacy protocols must be especially stringent. Privacy-by-design is an absolute necessity in this regard. Role-based access controls, anonymisation techniques, and encryption are essential for safeguarding data integrity and aligning with privacy regulations.
Putting in place a comprehensive data governance framework that takes the above into consideration is key to unlocking the full potential of AI while mitigating risks around data quality, ethics, and security.
“As AI continues to evolve, data governance frameworks must keep pace, ensuring that data integrity, accountability, and privacy are upheld. By integrating these principles, businesses can leverage AI responsibly, creating impactful and ethical solutions that drive meaningful insights and decision-making,” concludes Keyter.